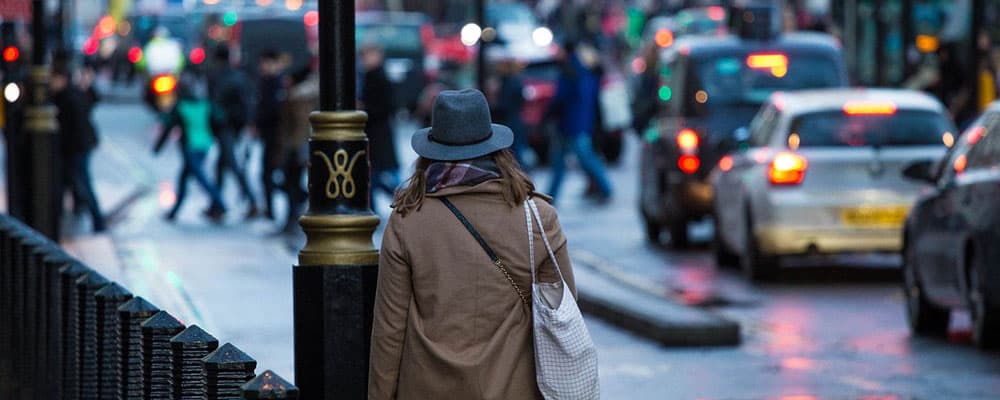
From ride-hailing apps and volatile gas prices to electric cars and (theoretically) autonomous vehicles, transportation behavior is rapidly changing. To properly plan for and manage our evolving transportation system, engineers and planners must keep pace with these changes. If managing transportation demand is important to your community, it’s not enough to follow the old pattern of creating new core analytics every 5-10 years to feed your models for any type of planning. For transportation demand management (TDM), which could be most profoundly affected by these new trends, the need for up-to-date, real, accurate data is even sharper.
In today’s evolving environment, effective TDM requires regular access to clean, up-to-date data. One problem that many planners face is that surveys and other traditional data-gathering methods simply cannot deliver high quality data at a frequent update cadence and affordable price.
Keep reading this blog post to learn all about TDM, and to find out how Big Data can help you maximize the impact of TDM strategies.
Data Challenges for Transportation Demand Management
Transportation demand management (TDM) is a set of strategies intended to reduce the demand for roadway travel, and especially for single-occupancy vehicles. Some of these strategies are aimed at reducing total travel demand, such as telework promotion, while others are designed to reduce peak period demand, like high-occupancy vehicle (HOV) lanes or ridesharing incentives.
TDM is an important tool for stakeholders to address a broad spectrum of agendas and concerns. And in today’s rapidly changing transportation environment, it requires vast amount of data gathered from an equally broad spectrum of sources.
A powerful strategy for addressing congestion, TDM helps planners convert vehicle trips to other travel modes, effectively removing cars from the roadways. In addition to reducing congestion, TDM may be used to help address the many other negative impacts of single-occupancy vehicles. According to the Federal Highway Administration, these negative impacts include:
- Poor air quality
- Reduced public and environmental health
- Less livable communities
- Dependence on oil
- Climate change and greenhouse gas emissions
Mandatory vs. Voluntary Strategies
Introduced in the 1970s, TDM has been an important concept in transportation, with new strategies introduced as a result of ongoing research efforts and technology advances. These strategies, according to the FHWA sourcebook, generally fall into two categories: mandatory and voluntary, or “carrot-and-stick.”
1. Mandatory (The “Sticks”)
These strategies aim to discourage driving by increasing the cost in terms of money, time or other factors. Road pricing programs, for example, may charge drivers higher fees to use certain roadways during peak periods. Managing parking availability and pricing are also effective ways to discourage drivers from using their cars.
Despite the effectiveness of these methods, “they are socially and economically controversial” according to the FHA sourcebook, “because they add to household and business expenses and the distribution of these expenses may be inequitable.”
There are examples of this type of strategy enacted in cities like London. Transport for London created a congestion charge in the downtown center during peak hours of the business week. This forced private automobile to pay a fee when driving in the area, and the city, ultimately, hopes this will eliminate the physical number of vehicles on the road.
2. Voluntary (The “Carrots”)
These strategies focus on encouraging alternatives to driving in single-occupancy vehicles by making these alternatives more feasible and less expensive than driving. One strategy for incentivizing ridesharing is the availability of high-occupancy vehicle (HOV) lanes, which may enable ride sharers to avoid costly congestion or tolls. Additional strategies include transit incentives, transit improvements and remote working.
As with the mandatory strategies, there are a plethora of cities that utilize ridesharing services and HOV lanes. In Washington D.C., a Casual Carpool Service called “Slugging,” has operated for over 40 years. It coordinates pick-up points for people who want to ride-share in and around the D.C. area. These types of services encourage commuters to not only share the monetary cost of driving, but also to eliminate the external costs that come with single-occupancy vehicles.
As with the mandatory strategies, there are a plethora of cities that utilize ridesharing services and HOV lanes. Where I live in the Washington, D.C. area, a Casual Carpool Service called “Slugging” has operated for over 40 years. It coordinates pick-up points for people who want to ride-share in and around D.C. This particular service was actually created by commuters themselves because riding alone meant they could not access faster-moving HOV lanes. Casual Carpoolers do more than an enjoy a less congested commute, however. They’re also helping to eliminate the external costs that come with single-occupancy vehicles.
Use Case: Transportation Demand Management with Big Data
For transportation demand management to be effective, planners must understand the full transportation system in detail, not just a few key intersections. In order to identify problems in the system, planners have particular need for detailed data on the following areas:
- Variable travel times (a sign of bad congestion)
- Very short trips
- Highly circuitous trips
Quality, updated data is also crucial for financing TDM projects. If planners do not have data that shows how many vehicle trips can be displaced by these projects, it is challenging to obtain financing for them. Instead, budgets are typically allocated to highway expansions or other projects that improve vehicle travel, rather than encouraging alternative transportation modes.
Big Data helps transportation professionals overcome these obstacles. First, the sheer volume of data available from mobile devices over very large swaths of territory allows planners to readily identify the specific locations that would most benefit from investment in TDM.
Scanning regions with Big Data reveals these “low hanging fruit” TDM locations much more effectively than traditional data collection methodologies. Big Data also allows transportation planners to quantify the relative volume of travel in each target situation, and thereby determine the impact of TDM projects on key metrics, such as level-of-service.
Example: Improving Pedestrian Access to a Metro Station
When people choose to drive very short distances (i.e.: under 2 miles) or take very complex, circuitous routes, they are typically reacting to an underlying multimodal mobility inconvenience. Removing that inconvenience can therefore make multimodal travel more convenient, reducing the number of short or circuitous trips.
Targeting such fixes in the right locations — that is, the ones with the most low hanging fruit in terms of trips — is more likely to reduce driving than focusing on neighborhoods where car trips are always long. One example is installing a pedestrian bridge because drivers cannot walk to the train station without crossing a major highway.
In northern Virginia, Big Data analyses of hundreds of road segments helped planners identify major problems with pedestrian access to the Van Dorn Metro station. Residents generally drove to the station, even though it was less than one mile away.
After using Big Data to identify the problem, one proposed TDM solution was improving sidewalks and adding a pedestrian bridge. These “carrot” incentives can be combined with “sticks,” such as the Metro station’s high parking costs. Together, these strategies are likely to divert the short, costly trips that residents were taking in their cars.
Beyond Transportation Demand Management
As we’ve discussed, Big Data is an essential resource for TDM, and for ultimately understanding — and changing — transportation behaviors. With the trend of Americans moving to metropolitan areas (for example, in 2013 metro areas saw their cities grow 0.9 percent in contrast to 0.7 percent in 2012 with 62% of millennials preferring these areas), such projects are important to manage the increased density. But it’s just the beginning. Here are seven other planning tasks and projects that can benefit from Big Data:
- Travel Demand Modeling
- Internal/External Studies
- Congestion Studies
- Project Performance Evaluations
- Performance Measures
- Detour Planning
- Public Transit Design and Expansion
What is your biggest challenge when it comes to transportation demand management? What tips or advice about Big Data would you offer to others? Do you have any other great examples of TDM projects that could use the help of Big Data? Let us know what you think by leaving a comment below.